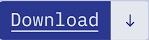
From this graph can be observed that erratic movement in search volume precedes a major stock crash.Īfter all adjustments of the data to eventually get relative daily data, which is relative to each other, the data visually looks as follows:įigure 2. A graph where the stock price of DJIA is plotted with red dots where a peak in search volume for "stock market" has been observed. There are also some peaks in the middle of a crash, but the peaks before the crash are quite indicative.įigure 1. As you can see, before a major stock market crash, there are usually some peaks to be observed. I plotted the DJIA stock price with indicators of peaks in the search volume for "stock market". To prove that there indeed is a correlation between Google Trends data (e.g.
Google trends stock prediction download#
To collect the stock price daily data for a specific ticker you want to predict, you have to download it from a website like Yahoo Finance, where you can download the historical data of any ticker. All this data will eventually be adjusted to be relative to each other, instead of only within its respective timespan.
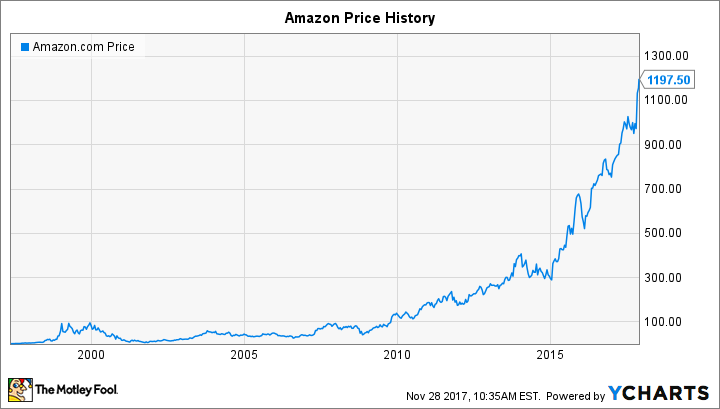
To collect the Google Trends daily data, you have to download all 6-month increments, 5-year increments, and 2004-present within the 2004-2020 timespan. Two datasets were needed for this project the Google Trends daily data for a specific keyword and the stock price daily data for a specific ticker.
Google trends stock prediction plus#
Comparison of the mean plus and minus 1 standard deviation of 10.000 random simulations, MLPClassifier algorithm, XGBoost algorithm and a buy-and-hold strategy. This was the reason why XGBoost still had such a large annual return (44.2%).įigure 9. However, XGBoost was still able to predict the stock market crash caused by the coronavirus. It tried to predict the small changes, which it ultimately failed at. XGBoost did not have the insight, which MLP did. Comparison of the mean plus and minus 1 standard deviation of 10.000 random simulations, MLPClassifier algorithm and a buy-and-hold strategy. Thus, for the most part, it held a buy-and-hold strategy, but during a stock market crash (like corona) or other, slightly bigger, changes, it performed well. This algorithm was very strong in identifying that it was impossible to predict the small changes in the market in between crashes. MLPClassifier performed very well on the test data. Because of the coronavirus, the stock market crashed, which could be a major source of profits for these algorithms.

A big contribution towards these insanely high annual returns was the coronavirus.
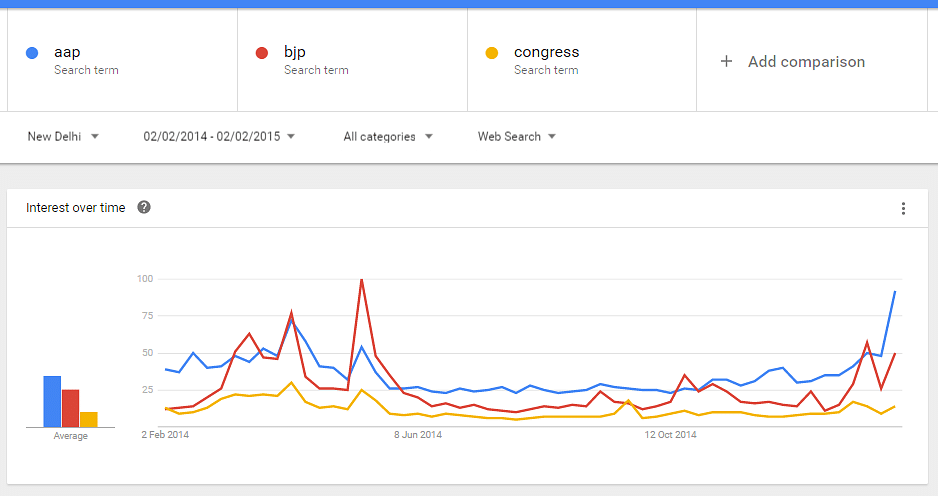
In contrast, MLPClassifier's best model got a 91.3% between 2008 and the present. The best annual return, which XGBoost got is 44.2%. The MLPClassifier clearly performed better than XGBoost. Two machine learning algorithms have been explored for this project: XGBoost and MLPClassifier. The purpose of this project is to combine this research with machine learning. In this research was found that the search volume for certain (financial) words are linked to the stock price of the Dow Jones Industrial Average stock price, and can in most cases predict a dip in the market. Eugene Stanley, "Quantifying Trading Behavior in Financial Markets Using Google Trends". The concept for this project came from research by Tobias Preis, Helen Susannah Moat, and H. The data used is downloaded from Google Trends.
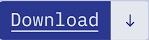